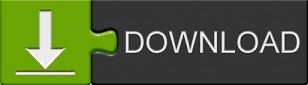
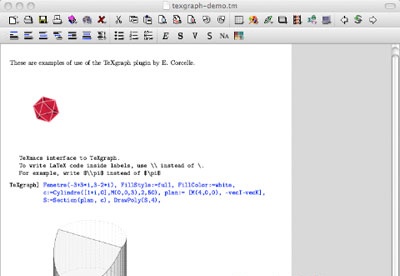
45 uL were transferred directly to NMR tube containing 5 uL of D2O with 100 uM DSS and mixed. Media samples were thawed at 4☌, then centrifuged at maximum speed for 15 minutes. Culture media was replenished on days 4, 6, 8, 11 after sampling. Was collected via pipetting as described in sample prep protocol document.Ĭultures were prepared with varied IL2 concentration, DMS concentration, and DMS surface densities as described in study design. Showing page 1 of 3 Results: 1 2 3 Next Showing results 1 to 100 of 235Ĭonditioned culture media (Miltenyi Texmacs media). Subject type: Cultured cells Subject species: Homo sapiens (Factor headings shown in green) mb_sample_id Edison, Theresa Kotanchek, Krishnendu Roy, and Wandaliz Torres-Garcia University of Georgia Georgia Institute of Technology University of Puerto Rico Mayaguezģ15 Riverbend Rd, Athens, Georgia, 30602, Source: This generalizable workflow and computational platform could be broadly applied to any cell-therapy manufacturing process to identify multivariate early CQAs and CPPs that are predictable of final product quality. Sequential, Design-of-Experiment (DOE)-based studies, coupled with a set of agnostic machine-learning framework, was used to extract multiple feature combinations from Day 4 to 6 media assessment, that were highly predictive (R2>90%) of the end-product phenotypes, specifically the total live CD4+ and CD8+ naïve and central memory T cells (CD63L+CCR7+ cells), and the CD4+/CD8+ T cell ratio. Using a degradable microscaffold-based T cell manufacturing process as an example, we developed an Artificial Intelligence (AI)-driven experimental-computational platform to identify a multivariate set of critical process parameters (CPPs) and critical quality attributes (CQAs) from heterogeneous, high dimensional, time-dependent multi-omics data, measurable during early stages of manufacturing and that are predictive of end-of-manufacturing product quality. However, the biological and logistical complexity of manufacturing a living product, including challenges associated with their inherent variability as well as uncertainties of process parameters, currently make it difficult to achieve predictable cell-product quality. Large-scale, reproducible manufacturing of therapeutic cells with consistent high quality is vital for translation to clinically effective and widely accessible cell-therapies for patients. Predicting T Cell Quality During Manufacturing Through an AI-based Integrative Multi-omics Analytical Platform A second validation experiment was performed with different values for culture parameters, using the same culture, sampling, and NMR analysis methods. Analysis of spectra resulted in a set of 20 features that was used in predictive modeling of T cell endpoints, along with culture parameters described above and cytokine data. Quantities and characteristics of activated T cells were assessed at the end of the culture period, and media was analyzed by 1H-NMR. Media of each culture of was sampled repeatedly over the course of a 14 day period.
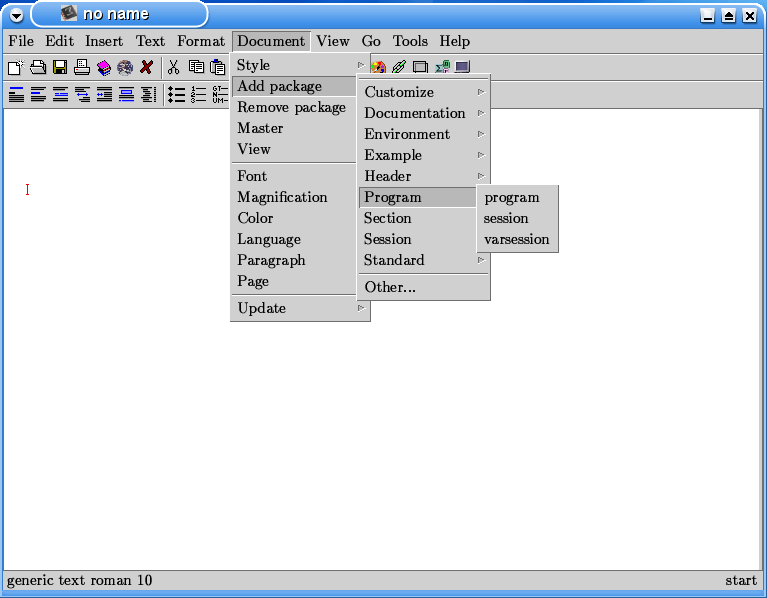
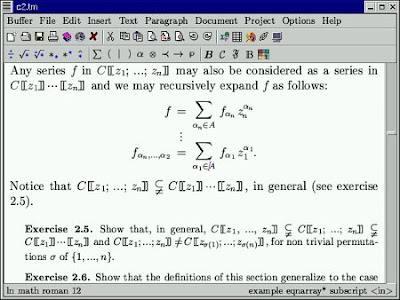
Magnetic bead cultures were expanded according to manufacturer’s instructions, while DMS cultures were expanded with varying DMS particle concentration, IL2 concentration, and antigen surface density on the particles, according to a design of experiments. Purified T cells from a single healthy donor were expanded in vitro with either magnetic beads or degradable micro-scaffold (DMS) particles. Design of Experiments for Maximizing T cell endpoints
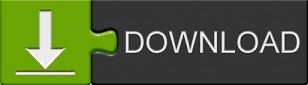